Efficient and Accurate Candidate Generation for Grasp Pose
Por um escritor misterioso
Last updated 25 fevereiro 2025

Recently, a number of grasp detection methods have been proposed that can be used to localize robotic grasp configurations directly from sensor data without estimating object pose. The underlying idea is to treat grasp perception analogously to object detection in computer vision. These methods take as input a noisy and partially occluded RGBD image or point cloud and produce as output pose estimates of viable grasps, without assuming a known CAD model of the object. Although these methods generalize grasp knowledge to new objects well, they have not yet been demonstrated to be reliable enough for wide use. Many grasp detection methods achieve grasp success rates (grasp successes as a fraction of the total number of grasp attempts) between 75% and 95% for novel objects presented in isolation or in light clutter. Not only are these success rates too low for practical grasping applications, but the light clutter scenarios that are evaluated often do not reflect the realities of real world grasping. This paper proposes a number of innovations that together result in a significant improvement in grasp detection performance. The specific improvement in performance due to each of our contributions is quantitatively measured either in simulation or on robotic hardware. Ultimately, we report a series of robotic experiments that average a 93% end-to-end grasp success rate for novel objects presented in dense clutter.
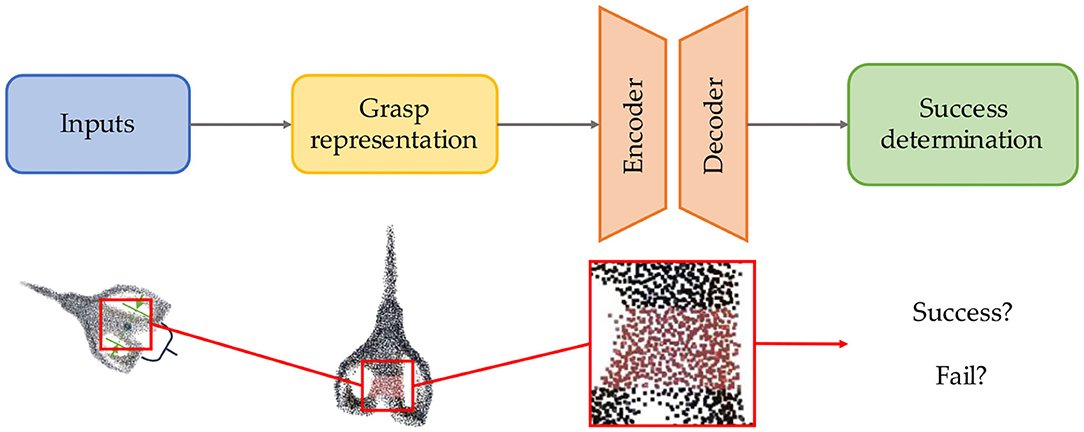
Frontiers Robotics Dexterous Grasping: The Methods Based on

Examples for grasp generation on single primitives. A sphere
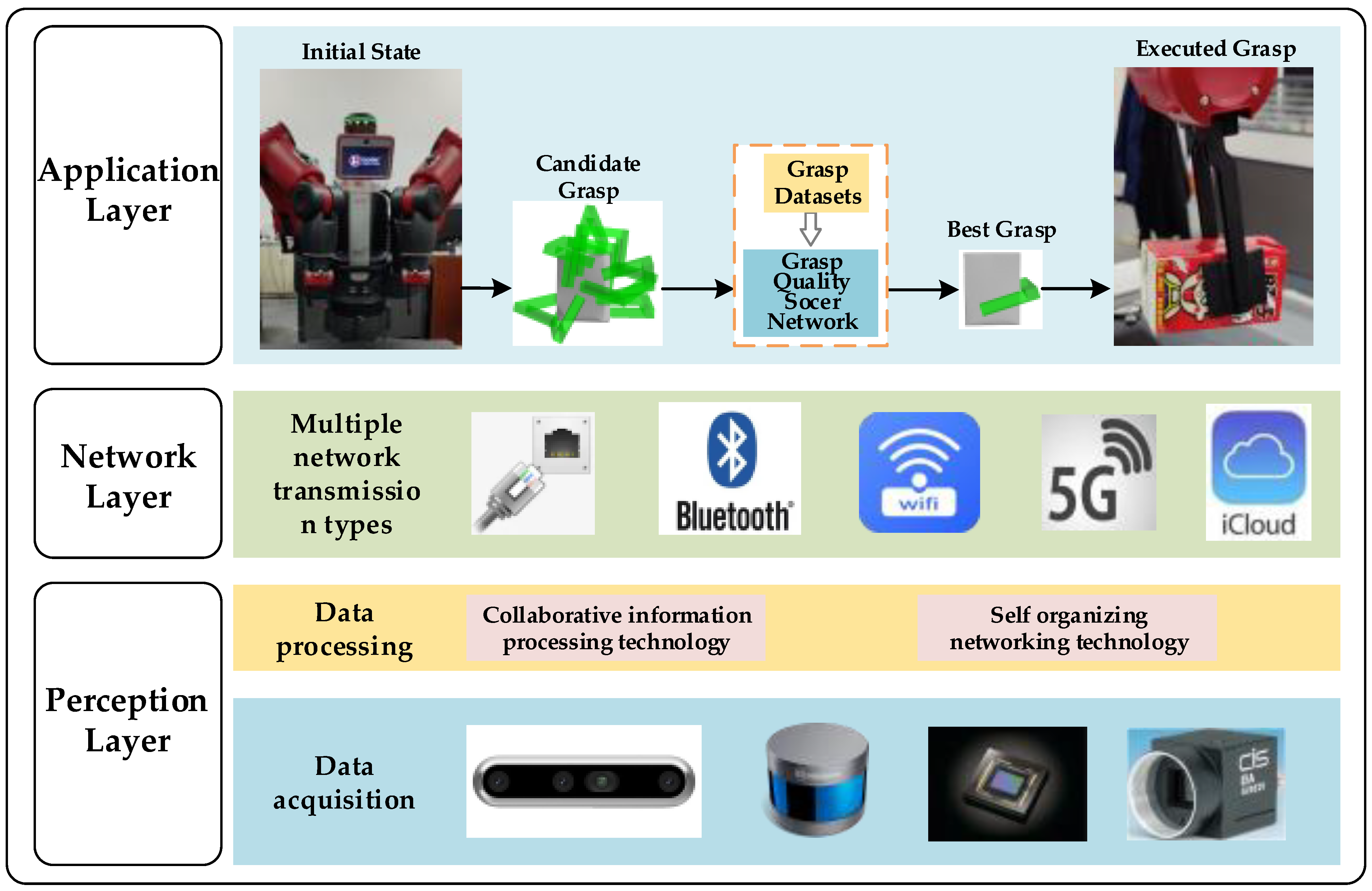
Micromachines, Free Full-Text

3D Grasp Pose Generation from 2D Anchors and Local Surface

The architecture of GraspCVAE. (a) In training, it takes both

Graphical model for robust parallel-jaw grasping of objects on a

Data-driven robotic visual grasping detection for unknown objects

Full article: A model-free 6-DOF grasp detection method based on
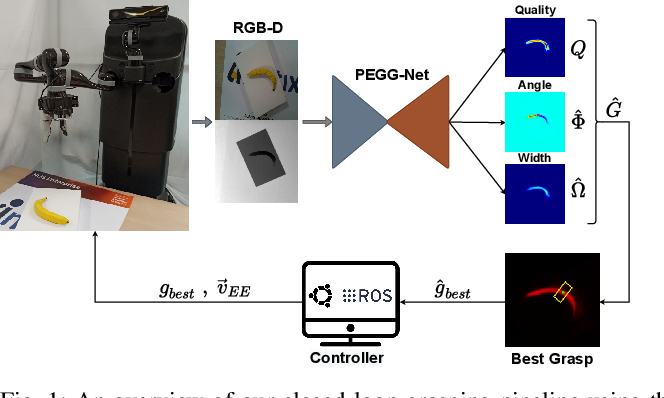
PEGG-Net: Background Agnostic Pixel-Wise Efficient Grasp

Grasp Pose Detection in Point Clouds - Andreas ten Pas, Marcus
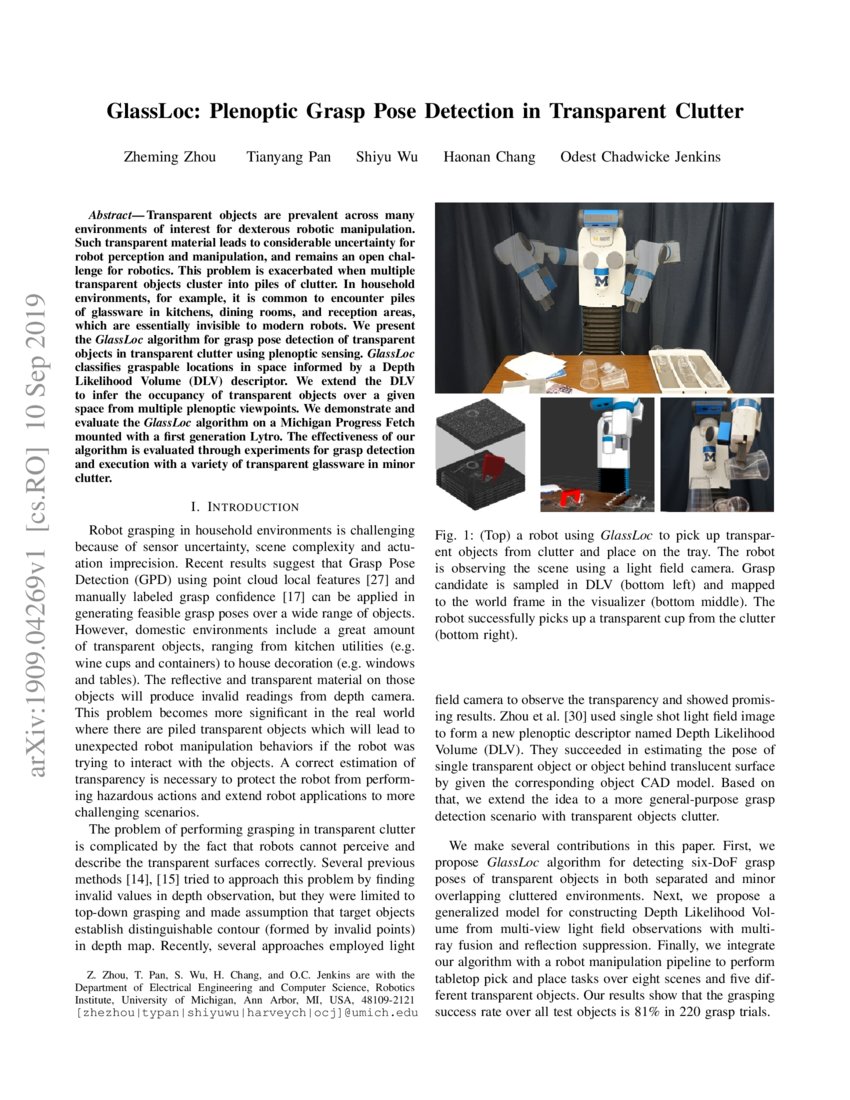
GlassLoc: Plenoptic Grasp Pose Detection in Transparent Clutter
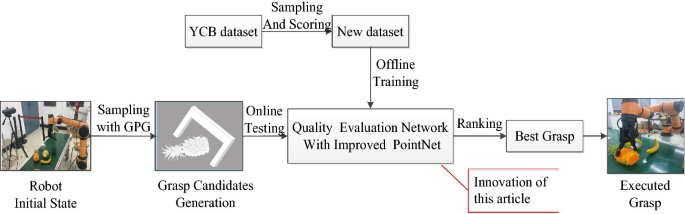
3D Grasping Pose Detection Method Based on Improved PointNet

PDF) Efficient and Accurate Candidate Generation for Grasp Pose

Dex-Net 2.0 Architecture. (Center) The Grasp Quality Convolutional

Efficient and Accurate Candidate Generation for Grasp Pose
Recomendado para você
-
Estamos à sua procura! Candidate-se!25 fevereiro 2025
-
Se Candidate, Mulher!25 fevereiro 2025
-
Se Candidate, Mulher! levanta R$ 1,2 mi para acelerar25 fevereiro 2025
-
MUVA on X: Atenção Pemba‼️ Estendemos o prazo das inscrições25 fevereiro 2025
-
Candidate-se ao - Instituto Nacional de Saúde - Moçambique25 fevereiro 2025
-
Colocamos ao menos uma mulher por dia no mercado de trabalho25 fevereiro 2025
-
Candidate-se ao Conselho Participativo Municipal25 fevereiro 2025
-
Candidate-se à segunda edição do Hackathon 100% Colaborativo25 fevereiro 2025
-
Relative gene expression (± SE) of 18 candidate genes by RT-qPCR25 fevereiro 2025
-
Candidate Search25 fevereiro 2025
você pode gostar
-
Joao Adibe Marques revela que é dono do famoso carro de Fórmula 125 fevereiro 2025
-
Hinata Hoshino, Watashi ni Tenshi ga Maiorita Wiki25 fevereiro 2025
-
Friends' Fan Week 2023 Merch25 fevereiro 2025
-
Ebros Gift Greek Mythology Gorgon Sisters Goddess Medusa with Wild Snakes Hair and Armored Scales Skin Bust Statue 10 Tall Temptation Seduction of25 fevereiro 2025
-
Whisper of the Heart - Wikipedia25 fevereiro 2025
-
Como dizer Para você também em inglês - English Experts25 fevereiro 2025
-
Fnaf 6 Pizzaria Simulator Download Five Nights at Freddys PT/BR Amino25 fevereiro 2025
-
League of Legends: Wild Rift - Requisitos y móviles compatibles25 fevereiro 2025
-
How To Get Advanced Skin Colors On Roblox25 fevereiro 2025
-
100+] Wild Rift Wallpapers25 fevereiro 2025